
In health research, there are many items or events that can be counted! For example, you might want to count the number of adverse events that occur when people take a medication, the number of times nurses wash their hands during their shift or the number of babies born in each month of the year. Frequency & Distribution of a Count VariableĬount variables refer to those that simply tally the number of items or events that occur. FREQUENCY DISTRIBUTION FOR CATEGORICAL RESPONSES' įigure 13.1 Frequency Distribution For a Categorical Response Variableįrequency distributions are useful in describing variables, helping to identify errors (impossible values) and outliers, assessing how well a continuous variable fits the normal distribution, or to test hypotheses using specific statistical tests such as using a chi-square test to evaluate categorical variables. OPTIONS PAGESIZE=55 LINESIZE=120 CENTER DATE Table 13.1 Frequency Distribution For Categorical Responses The Category Label is presented on the x-axis, and the number of responses-frequencies for each response are presented on the y-axis. The following is an example of a table showing a frequency distribution for a set of responses to a categorical variable ranging from 1 to 5, and a graphical representation of the frequency of responses in each category. 1, 2, 3, 4, or 5) – in other words, how frequent each score appeared within the total set of scores. You may consider reviewing the number of respondents in your sample had each possible score (i.e. You might also consider responses to an anxiety questionnaire scored on a Likert scale (using discrete scaled scores) ranging from 1 to 5. For example, you might look at the number of patients presenting to the Emergency Department for different reasons: cardiovascular concerns, accidents, infections, reported symptoms. For example, datasets that include only discrete scores ranging from 1 to 5 would not be expected to fit a normal distribution curve but would rather be compared to a categorical distribution curve – like the chi-square distribution or the Poisson distribution.ĭistributions can be obtained by counting the number of events that occur or how many participants in a sample have a specific score on a questionnaire or measure (i.e., counting frequencies). What you may not realize is that distributions other than the normal distribution are also used in statistic analyses and that datasets can take the shape of these other distributions. If you studied statistics previously you are most likely familiar with the normal distribution or bell curve. Distributions are used to describe the organization or shape of a set of scores or values for a particular variable.

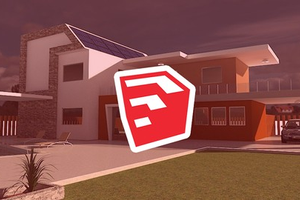
It could also be the number of times an electrical signal with a given level of energy-intensity is recorded.Ī distribution shows the relative frequencies of each possible value or category for a variable.


It could be, for example, the number of people with brown hair, the number of children in a family, the number of deaths in a hospital. While we won’t go into detail about the differences between frequentist and Bayesian statistical approaches, it is important to recognize that frequencies play a key role in the approach that we are demonstrating here but that a frequentist approach is not the only way to analyze your data.Ī frequency is simply the number of times something happens. An alternative approach is called the Bayesian statistical analysis which relies on probabilities. In fact, this approach is often referred to as “frequentist statistics” because it relies on frequencies to make inferences about the data. Frequencies and distributions are important concepts in the quantitative analysis of data that underlie the overall statistical approach covered in this book. These simple but powerful analyses enable you to examine your data and identify patterns including the shapes and distributions of data, missing values, and outliers. Throughout this text, we will focus on using frequency analysis and descriptive statistics. Goodness of Fit and Related Chi-Square Tests 13 Frequency Distributions 13.1 Analyzing Distributions of Data
